As fraud rates climb, with losses projected to reach more than USD 43 billion worldwide by 2028, fraud detection and prevention have become increasingly critical for marketplaces and platforms, regardless of their size and business model.
This article looks into why businesses need fraud detection techniques, focusing on who's at risk, what the challenges are, and how to set up good fraud prevention to stop fraud in real time.
What business models do fraudsters target?
Fraudsters usually consider two criteria when targeting businesses. They are looking for a profitable model with large volumes of sales. Blending in among thousands or millions of users and transactions is easier.
They also look for any loopholes. Even if there isn’t a lot of money changing hands there, any space with security lapses is an easy target for them.
When it comes to different industry sectors where marketplaces and platforms operate, it's clear that no business is immune to the risk of fraud. Common threats include payment fraud and account takeover fraud, wherein fraudsters break into accounts and make unauthorized transactions. Similarly, chargeback fraud leads to financial losses and harms businesses' relationship with card networks. Here are the industries most targeted by these threats:
- Product marketplaces
- Digital goods
- On-demand marketplaces
- Financial platforms
- Travel platforms
- Gig economy
- B2B platforms
Top fraud types that platforms need to watch
When we talk about fraud, it's important to also understand the level of interaction of your users with your website and app. There are three critical stages that need extra attention for fraud: user registration, checkout, and the post-payment stage.
Login and registration - risk at the front gate
At this stage, the intent is either to infiltrate a legitimate account to misuse it or to create a new, fictitious one - usually through identity theft - as a vessel for fraudulent activities. The fraud types that can occur are:
- account takeover: fraudsters break into existing genuine accounts, gaining unauthorized access to carry out malicious activities.
- account opening fraud: new fake accounts are created for the sole purpose of committing fraud.
- multi-accounting: often aimed at exploiting promo deals or policies, this involves creating multiple accounts by a single user or entity.
Checkout - risk during transaction attempt
When the customer is about to make a purchase, you need to confirm the transaction is legitimate—that it's truly the customer making the transaction. Various types of fraud occur at this stage, such as:
- CNP fraud: card-not-present fraudulent transactions are completed using payment card information without the physical card being presented.
- Promo and policy abuse: fraudsters exploit promotional offers or policy loopholes for unauthorized gain, often by creating multiple accounts or misusing return/refund policies.
- Reseller fraud: unauthorized selling of a company's products or services, often at lower prices, leading to potential revenue losses.
Post-payment - risks beyond the purchase
After fulfilling an order, the outcome is uncertain. You might soon get a review, a return request, or a chargeback notification. In the most unfortunate situations, platforms have to deal with:
- Chargebacks: the legitimate action of a user filing a dispute with their issuer claiming their money back for various reasons, like 'order not fulfilled'.
- Friendly fraud or first-party misuse: the same process as for the legit chargeback, but under false claims - customers are trying to exploit the chargeback process to get something for free.
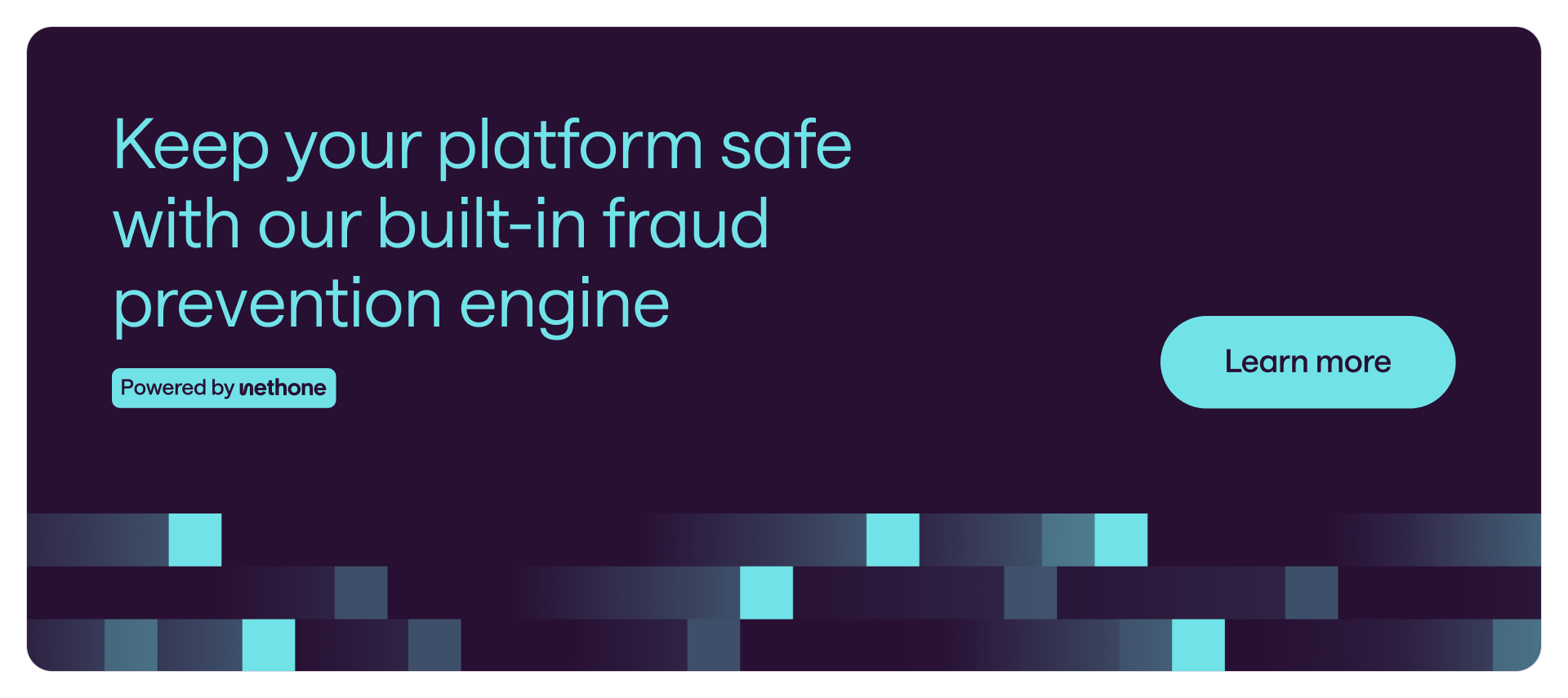
Tactics that bypass fraud detection
Fraudsters use social engineering, card testing, network anonymization, credential stuffing, and bots to get into genuine accounts and make unauthorized transactions. They are not intimidated by robust protective measures. They try until they manage to get in. That’s why a completely fraud-free environment is far from reality.
Social engineering
Fraudsters manipulate users into revealing their account details or other sensitive information by posing as trustworthy entities, such as a customer support representative or a friend. They usually approach their victims via email or phone, and lately, they've started misusing Remote Access Tools (RATs).
Fraudsters pretend to be customer service reps or sellers on platforms. They might reach out to buyers, saying there's a problem with their order and pretending to be the seller. Then, they trick the buyer into installing a RAT by claiming it will help solve their issue faster. This trick gives the fraudster access to the buyer's computer or device and any information on it - a tactic similar to the one used in 'The Beekeeper' movie.
Card testing
Fraudsters steal credit or debit card details and use this information to make transactions. They often buy this data from the dark web. Before using a card, fraudsters need to make sure it's still good and has money in the account. They have their own ways of checking the card's validity without alerting the real owner.
Network anonymization
Fraudsters hide their tracks, like their real IP address and their location, by using VPNs, residential proxies, or TORs. Skilled fraudsters use illegal VPN services and proxies that are tough for fraud fighters to detect.
Credential stuffing
Fraudsters take leaked or stolen usernames and passwords and try them on various accounts, betting on the chance that people have used the same login details on different sites.
Bots
Fraudsters use bots to mimic human actions. In the login and registration stage, bots fill out forms, create accounts, and sign up for services using fake identities. In the checkout stage, with bots, fraudsters can quickly buy up all available items on a marketplace, like limited edition products, to commit reseller fraud afterward.
The challenges and flaws in fraud detection
Fraud prevention is also about finding the balance between helping a business grow and keeping it safe from fraud. You don't have to choose between the two but should aim to balance both objectives.
We usually identify two approaches in this matter:
1. The goal is to stop as much fraud as early as possible. This approach operates on the idea that it's better to decline a few legitimate transactions, which might slightly lower conversion rates than to suffer the consequences of fraud.
2. The goal is to accept as many transactions as possible to create a frictionless customer experience, even if it means accepting the risks of fraud. This can increase the checkout experience and sales, but it's a bet that could lead to fraud issues down the line.
Regardless of the approach, the most common challenges that businesses struggle with are false positives and declines, manual reviews, 3DS friction, fraud rates, and the overall user experience.
False positives and false declines
A fraud prevention system that is too strict can block genuine customers. Saying no to a payment attempt can both help and hurt. It's good because it can stop fraud, like when someone tries to use a stolen card. But it's also risky because you might accidentally block a real customer from buying.
Manual reviews
If you rely solely on manual processes, fraud may slip through, or the false positives rate might increase, especially if you experience a high volume of account openings or transactions. It’s nearly impossible to detect with high precision enough fraud coming in.
3DS authentication
3DS authentication is useful, but consumers might feel it's not their job to secure a transaction, so why would they do this extra step? The key is to find a way to make things easy for your consumers while keeping everything safe. The best way to do this is only to add extra steps when there's a real need and exempt when the transaction looks safe.
Fraud rates and other KPIs
The goal of any fraud management team is to lower the overall fraud rate of the business. The challenge here lies in addressing this goal against the other challenges related to false positives and user experience.
Beyond fraud rates, metrics like chargebacks, abandonment, authorization, and 3DS rates are also crucial and vary with business size and model. Small marketplaces might need simpler reporting, while big companies track more metrics.
Fraud detection technologies
The best approach to detecting fraud varies across businesses, taking into account the needs and challenges each faces, as well as the scale and nature of transactions. A marketplace with a relatively small number of transactions may lean towards a system based on pre-defined rules, whereas platforms that handle billions of transactions annually likely require increased protection, including bespoke machine learning models built by experienced data scientists.
Ultimately, achieving the most precise fraud detection without disrupting the customer experience is the goal. Here's what you need to strike the balance.
Machine learning
Machine learning (ML) models analyze patterns in countless transactions in real time. As they process more data, they become better at detecting any suspicious behavior, adapting their strategies on the fly without needing manual updates.
ML can also help optimize 3DS exemptions. They recognize transactions that are likely risk-free, so you can allow good users to go through without extra security checks while triggering 3DS only for risky transactions.
Rules-based system
Rules engines are a great tool for fraud management, especially when you create granular, in-depth rule sets to increase fraud detection precision and reduce false positives. A well-designed rules engine should enable you to:
- Easily customize and edit rules at any time
- Simulate rules using historical data to assess its success before going live
- Quickly adapt rules in response to changing fraud patterns
Hybrid system
A hybrid system combines machine learning models with static rules. While you have the flexibility to implement rules aligned with your KPIs, we advise allowing the machine learning engine to have the ultimate say in decisions.
Risk detection
Whether it operates on rules or machine learning, a fraud management tool must continuously collect and analyze data related to user transactions. Such data can include how often a user shops at a particular e-commerce site, their unique way of moving around the website, specifics about their device like the processor, the unique ID of the device, and more.
The volume of data is critical, but so too are the data sources used. Fraudsters use various methods in their attempts to outsmart anti-fraud systems. They spoof web browsers, operating systems, and devices to hide their tracks. Gathering data around the user helps in detecting unusual patterns and enables more accurate fraud prevention, especially in cases of credit card fraud detection or account takeover.
Reputation scores
Reputation scores or risk scores are like grades that tell how likely a user might be involved in fraud. When a fraud prevention system checks a user, it takes into account both information given by the user (like their phone number) and information it collects itself (like the user's IP address or device ID. This process of data consolidation, often referred to as data enrichment, helps the system detect the risk of fraud.
The power of network effect
User-generated data becomes more valuable when shared across a larger system. A wider network of information helps the system continuously learn and get better at spotting fraud. The more users a system protects, the more knowledge it gains, and the better and quicker it can react to the new ways fraudsters operate.
The bottom line
Whether you're considering a new solution or looking to complement the existing one, the guiding principles are the same:
Fighting fraud is also about teamwork. Your feedback and input are just as important for system learning as the expertise and research provided by the solution provider. Together, you can discover emerging fraud and new strategies and solutions.
Transactions on mobile are growing, and most likely, you’re already seeing this. Look for a solution that is equally powerful on mobile-native apps and on web browsers.
The future leans heavily towards AI, but rule-based systems are here to stay. The best approach mixes both with a good helping of human expertise, especially from data scientists.
Keep up with fraudsters’ tactics while protecting your good users! Get in touch with us to learn how.